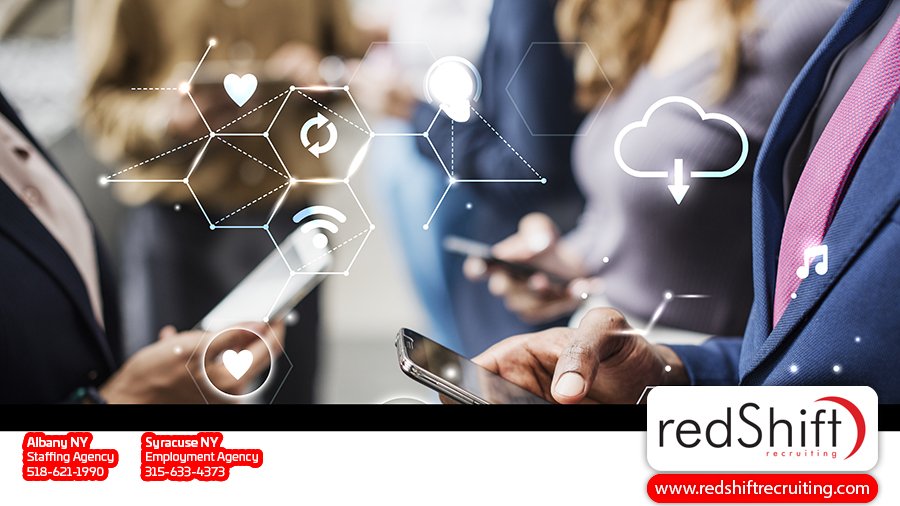
The Role Of Data Science In Machine Learning, Deep Learning, And Ai
Introduction
Are you curious about the fascinating world of data science and its pivotal role in machine learning, deep learning, and artificial intelligence? Prepare to embark on a thrilling journey where you will unravel the mysteries behind these cutting-edge technologies.
In this article, we will delve into the intricate web of data science and explore how it intertwines with the realms of machine learning, deep learning, and AI.
As you dive deeper into this article, you will gain a profound understanding of data science's significance in driving advancements in technology. Data science is not just a buzzword; it is a discipline that empowers us to extract valuable insights from vast amounts of raw data. By employing analytical techniques and statistical models, data scientists transform complex datasets into meaningful information that fuels innovation.
So why is data science so crucial for machine learning, deep learning, and AI? Well, my friend, these technologies heavily rely on patterns and correlations found within massive datasets. By utilizing powerful algorithms developed by data scientists, machines can learn from experience and make intelligent decisions without explicit programming. Deep learning takes this concept even further by mimicking the neural networks of our own brains. And at the pinnacle stands artificial intelligence – an ever-evolving field that aims to create machines capable of human-like intelligence. To unlock their true potential, all three depend on the expertise of data scientists who harness the power hidden within troves of structured and unstructured data.
Now that your curiosity has been piqued about the interplay between data science and these transformative technologies – machine learning, deep learning, and AI – let us embark on a captivating exploration through their intricacies. With each step along this path, you'll discover how various tools and techniques shape real-world applications across different industries. So strap yourself in as we navigate through case studies showcasing impactful use cases while also peering into future trends that promise even greater possibilities for both data science aficionados and those merely dipping their toes into its vast ocean of possibilities.
Introduction to Data Science
Data science is like the key that unlocks the treasure trove of insights hidden within vast amounts of data, bringing machine learning, deep learning, and AI to life. It plays a pivotal role in these fields by providing the necessary tools and techniques to analyze, process, and understand data.
A data scientist is like a detective, using their analytical skills to uncover patterns, trends, and relationships within complex datasets.
In the realm of machine learning and deep learning, data science acts as the foundation upon which these technologies are built. Without proper data analysis and processing, it would be impossible to train accurate models or make informed predictions. Data scientists leverage various statistical methods and algorithms to extract valuable information from raw data. They also employ advanced techniques such as feature engineering and dimensionality reduction to optimize model performance.
Furthermore, in the field of AI, data science enables machines to learn from experience and improve their performance over time. By utilizing large datasets and applying advanced algorithms, data scientists can create intelligent systems that can understand natural language, recognize objects in images, or even play complex games at a high level.
The role of data science extends beyond just analysis; it encompasses the entire data science process from problem formulation to deployment. Data scientists engage in projects that involve collecting relevant data sources, cleaning and preprocessing the data for analysis purposes, building models based on this processed information using machine learning algorithms or deep neural networks tailored specifically for each task at hand before finally deploying them into production environments where they can be used by others who may not have any expertise themselves but still need some help understanding what's going on behind those black boxes we call 'AI' today!
Understanding Data Science and Its Role
With a comprehensive understanding of the intricacies involved, one can delve into the intricate realm where data science and artificial intelligence converge. Data science plays a crucial role in machine learning, deep learning, and AI by providing the necessary tools and techniques to analyze vast amounts of data.
Data scientists are equipped with the expertise in computer science, statistical analysis, and mathematical skills that enable them to extract valuable insights from both structured and unstructured data. They employ various methods such as data mining, data analytics, and data visualization to uncover patterns and trends that would otherwise go unnoticed. By developing sophisticated algorithms and models, they create systems that can process large volumes of information efficiently.
To truly understand the importance of data science in AI, it is essential to recognize its key responsibilities. Data scientists not only collect and organize data but also clean and preprocess it to ensure its quality before analysis. They use statistical techniques to identify correlations between variables and make predictions based on these relationships. Additionally, they develop accurate models using machine learning algorithms that can learn from past experiences and adapt their behavior accordingly. With their expertise in handling complex datasets, they contribute significantly to improving the accuracy of AI systems.
In summary:
Data scientists possess the necessary skills in computer science, statistics, mathematics, etc., which allow them to analyze vast amounts of structured and unstructured data.
They utilize various techniques such as data mining, analytics, visualization to extract valuable insights from the collected information.
Their role involves cleaning and preprocessing the collected data for accurate analysis.
By developing sophisticated algorithms and models through machine learning techniques, data scientists enhance the accuracy of AI systems.
If you have an inclination towards working with large datasets or have a passion for problem-solving using advanced analytical tools like Python or R programming language; if you find joy in visualizing complex information or predicting future outcomes based on patterns; if you enjoy exploring diverse industries such as healthcare or finance while leveraging your analytical mindset - then you have the potential to become a data scientist. With the growing demand for professionals in this field, pursuing a career as a data scientist can open doors to exciting opportunities and allow you to make significant contributions to the world of AI and machine learning.
So, if you're ready to embark on this rewarding journey, start by developing your statistical and mathematical skills, gaining expertise in data analysis techniques, and familiarizing yourself with different data models. By honing these abilities, you can pave your way towards becoming a successful data scientist.
Machine Learning, Deep Learning, and AI
By delving into the realm where technology and innovation converge, we can unlock the incredible potential of machine learning, deep learning, and AI.
Data scientists play a crucial role in this domain by developing and deploying machine learning models that can analyze vast amounts of data to extract meaningful insights. They use their expertise in statistics, mathematics, and programming to create algorithms that enable machines to learn from data and make accurate predictions.
Data scientists rely on various techniques such as predictive modeling and predictive analysis to build these machine learning algorithms. They work closely with data engineers who are responsible for ensuring the smooth flow of data from different sources through processes like data acquisition and transformation. Additionally, they utilize data visualization tools to present complex quantitative data in a visually appealing manner, making it easier for decision-makers to understand and act upon.
Deep learning is a subfield of machine learning that focuses on training artificial neural networks with multiple layers. Data scientists leverage deep learning techniques to solve more complex problems by enabling machines to learn abstract representations of the input data. This allows them to perform tasks such as image recognition or natural language processing with remarkable accuracy.
The role of data science in machine learning, deep learning, and AI cannot be overstated. Data scientists are at the forefront of developing advanced algorithms and models that enable machines to learn from vast amounts of data. Their expertise in areas such as predictive modeling and deep learning empowers organizations across various industries to uncover valuable insights from their data and make informed decisions.
Data Science Techniques and Tools
Utilizing cutting-edge methodologies and advanced technologies, data scientists and machine learning engineers employ a wide array of techniques and tools to extract valuable insights from complex datasets. With their expertise in statistical analysis, they're able to perform exploratory data analysis to gain a deeper understanding of the data.
By identifying patterns, trends, and outliers, they can make informed decisions on which machine learning techniques are most suitable for the task at hand.
To facilitate their work, data scientists rely on various data science tools. They utilize data pipelines to streamline the process of collecting, cleaning, and transforming raw data into a format that's ready for analysis. These pipelines ensure that the data is consistent and accurate throughout the entire workflow.
Additionally, data storage solutions enable efficient access to large volumes of data while maintaining its integrity.
Data visualization plays a crucial role in conveying insights effectively. Data scientists use visualization tools to create informative charts, graphs, and dashboards that allow stakeholders to easily interpret complex information. Qualitative analysis techniques are also employed to extract meaningful insights from unstructured or textual data.
The field of data science encompasses a range of techniques and tools that enable experts to analyze complex datasets effectively. By leveraging statistical analysis, exploratory data analysis, and qualitative analysis methods along with utilizing various tools such as data pipelines, storage solutions, and visualization tools, professionals in this field provide valuable insights for decision-making processes in machine learning applications.
Real-World Use Cases and Impacts
One fascinating statistic that illustrates the real-world impacts of data science is that in 2019, Walmart reported a 10% increase in online sales after implementing personalized product recommendations based on customer browsing and purchase history. This demonstrates the power of data science techniques and tools in driving business outcomes.
By leveraging big data and applying predictive models, skilled data scientists were able to extract valuable insights from the vast amount of customer information available. These insights enabled Walmart to create personalized product recommendations tailored to each individual customer's preferences, resulting in higher sales and improved customer satisfaction.
Data science professionals play a crucial role in transforming raw data into actionable insights. Data analysts are responsible for extracting meaningful information from large datasets, while data engineers ensure the smooth flow and storage of data across systems. Working together as part of a data science team, these professionals collaborate to uncover patterns and trends hidden within the data.
By harnessing their expertise, businesses can make informed decisions that drive growth and competitive advantage. In an era where information is abundant but scattered, it's the skilled data scientists who possess the ability to turn this wealth of raw material into valuable knowledge that propels organizations forward.
Future Trends in Data Science and AI
Imagine a future where data scientists are at the forefront of groundbreaking technological advancements, seamlessly integrating cutting-edge innovations in data analytics with various fields to revolutionize industries and shape the AI landscape.
In this future, data science team members will play a crucial role in bridging the gap between traditional disciplines and machine learning. They'll collaborate with experts from different domains, such as healthcare, finance, and manufacturing, to identify opportunities for applying data science techniques and create innovative solutions.
Data science programs will evolve to equip professionals with the necessary skills to navigate this ever-changing landscape. Aspiring data scientists will learn not only programming languages but also how to work effectively within interdisciplinary teams. Data analysts will gain expertise in complex quantitative algorithms while business analysts will develop a deeper understanding of data architecture. Data science managers will emerge as leaders who can effectively communicate the value of data-driven insights and guide their teams through the entire data science lifecycle.
This future envisions a world where organizations understand the importance of investing in their data professionals and fostering a culture that values analytical thinking. As industries continue to generate vast amounts of data, there will be an increasing demand for skilled individuals who can extract meaningful insights from this information overload. By embracing these trends, businesses can unlock new opportunities for growth and remain competitive in an AI-driven world.
Frequently Asked Questions
What are the ethical implications of using data science in machine learning, deep learning, and AI?
Using data science in machine learning, deep learning, and AI raises important ethical implications that need to be carefully considered. One of the key concerns is the potential for bias in the data used for training these algorithms. Since machine learning models learn from historical data, if that data contains biases or reflects societal inequalities, it can perpetuate and amplify those biases in its predictions. This can lead to unfair outcomes or discrimination against certain groups.
Another ethical consideration is privacy and security. Data scientists have access to vast amounts of personal information, and there is a risk of misuse or unauthorized access to this data. Protecting individuals' privacy rights while harnessing the power of data science for AI development is crucial.
Additionally, transparency and accountability are essential when using these technologies. Users should have an understanding of how decisions are made by AI systems so that they can trust them and hold them accountable when necessary. Ensuring fairness, privacy protection, and accountability are fundamental aspects of addressing the ethical implications associated with using data science in machine learning, deep learning, and AI systems.
How can data scientists ensure the accuracy and reliability of the data used in their models?
To ensure the accuracy and reliability of the data used in your models, imagine you're a detective on a mission to solve a complex case. Just as a detective carefully examines every piece of evidence, you must scrutinize your data with precision and attention to detail.
Start by thoroughly understanding the source of your data and its potential biases or limitations. Use statistical techniques to identify and remove outliers that could skew your results.
Validate your data through cross-validation or independent verification, like seeking second opinions from other experts. Embrace transparency by documenting your data preprocessing steps, ensuring reproducibility for others who may want to validate or build upon your work.
Lastly, remember that no dataset is perfect; it's an ongoing journey where continuous monitoring and adaptation are key to maintaining accuracy and reliability in your models. By approaching the task as a dedicated investigator committed to uncovering the truth, you can forge trustworthy paths towards advancing machine learning, deep learning, and AI while satisfying our inherent desire for belonging through reliable insights.
What are the limitations and challenges of implementing data science techniques in real-world scenarios?
Implementing data science techniques in real-world scenarios can present several limitations and challenges. One major limitation is the availability and quality of data. In many cases, real-world data may be incomplete, inconsistent, or biased, leading to inaccurate models and predictions.
Additionally, the sheer volume of data generated by various sources can overwhelm traditional data processing methods, making it difficult to extract meaningful insights efficiently.
Another challenge lies in the complexity and interpretability of machine learning models. While these models can provide highly accurate predictions, understanding how they arrive at those predictions is often elusive. This lack of transparency makes it challenging to trust and explain the decisions made by these models in critical applications such as healthcare or finance.
Lastly, there are ethical considerations surrounding privacy and fairness that need careful attention when implementing data science techniques in real-world scenarios. It's crucial to ensure that sensitive personal information is protected and that algorithms don't perpetuate biases or discrimination unintentionally.
Overcoming these limitations requires a combination of domain knowledge, rigorous methodology, robust validation processes, and a commitment to continuously improving and refining models based on feedback from real-world applications.
By addressing these challenges head-on, data scientists can harness the power of data science to make valuable contributions in various fields while ensuring accuracy and reliability for their stakeholders.
What are the potential risks and consequences of relying too heavily on automated decision-making powered by data science?
Relying too heavily on automated decision-making powered by data science can have potential risks and consequences. For example, imagine a scenario where a large-scale financial institution uses an algorithm to determine creditworthiness for loan applications. This algorithm relies solely on historical data to make its decisions, without taking into account any contextual factors or individual circumstances.
As a result, the algorithm may disproportionately reject loan applications from certain demographic groups, leading to biased outcomes and perpetuating existing inequalities. In this case, the consequence of relying too heavily on automated decision-making is the reinforcement of systemic discrimination and exclusion.
It's crucial to strike a balance between automation and human judgment in order to mitigate such risks and ensure fair and ethical outcomes for all individuals involved.
How can businesses effectively integrate data science into their existing workflows and processes?
To effectively integrate data science into your existing workflows and processes, you need to start by understanding the specific needs and goals of your business. Begin by identifying areas where data science can bring value and enhance decision-making.
This could involve analyzing customer data to gain insights into their preferences and behaviors, optimizing supply chain operations through predictive analytics, or using machine learning algorithms to automate repetitive tasks.
Once you have identified these opportunities, it's crucial to build a strong foundation for data-driven decision-making by investing in the necessary infrastructure, tools, and talent. This may include implementing robust data management systems, training employees on data literacy and analysis techniques, and fostering a culture that values experimentation and continuous improvement.
By integrating data science seamlessly into your workflows, you can unlock the full potential of your business's data assets and drive innovation while making more informed decisions that lead to sustainable growth.
Conclusion
In this data-driven future, organizations will witness a transformative shift as data scientists become the driving force behind groundbreaking technological advancements, reshaping industries and propelling society into an era of unprecedented innovation. As the demand for data-driven decision making continues to rise, individuals who possess the skills and knowledge in data science will hold a significant advantage in shaping the future of machine learning (ML), deep learning (DL), and artificial intelligence (AI).
Here are some key reasons why you should explore data science and leverage its power:
Data Science Courses: By enrolling in data science courses, you can gain a comprehensive understanding of statistical analysis, programming languages like Python or R, and machine learning algorithms. These courses provide you with the necessary foundation to excel in the field.
Data Summarization and Reporting: With expertise in data science, you'll be able to effectively summarize complex datasets into meaningful reports. This skill is crucial for businesses looking to make informed decisions based on accurate information.
Building Complex Quantitative Algorithms: Data scientists have the ability to develop intricate algorithms that can process vast amounts of data efficiently. This capability is essential for creating sophisticated ML models and DL architectures.
Analyzing and Interpreting Data: By honing your skills in analyzing and interpreting various types of data, you can extract valuable insights that may contribute to solving real-world problems. The ability to understand patterns within datasets is vital for developing AI systems.
Data Visualizations: Being able to present complex information visually through graphs or charts helps stakeholders easily comprehend large sets of data. As a proficient data scientist, you'll be skilled at creating compelling visualizations that convey meaning effectively.
By diving into the world of data science, not only will you learn how to manipulate and analyze massive amounts of information but also extract meaningful insights from it. You'll gain expertise in areas such as statistical analysis, programming languages like Python or R, building complex quantitative algorithms, summarizing and reporting data, analyzing and interpreting different types of data, creating visually appealing visualizations, and staging data for ML and DL models.
Embracing data science is a pathway to becoming a driving force in the future of technology, allowing you to contribute to advancements in ML, DL, and AI that will shape industries and society as a whole. So why wait? Start your journey into the fascinating world of data science today!